TOKEN MERGING: YOUR VIT BUT FASTER
Abstract
Token Merging(ToMe) gradually combines similar tokens in a transformer using a general and light-weight matching algorithm that is as fast as pruning while being more accurate.
Introduction
Yet, token pruning has several disadvantages:
the information loss from pruning limits how many tokens you can reasonably reduce;
current methods require re-training the model to be effective (some with extra parameters);
most cannot be applied to speed up training; and several prune different numbers of tokens depending on the input content, making batched inference infeasible.
TOKEN MERGING
Strategy
In each block of a transformer, we merge tokens to reduce by r per layer. Note that r is a quantity of tokens, not a ratio.
Importantly, we reduce rL tokens regardless of the image’s content.
We apply our token merging step between the attention and MLP branches of each transformer block. This is also in contrast to prior works, which tend to place their reduction method at the beginning of the block instead. Our placement allows information to be propagated from tokens that would be merged and enables us to use features within attention to decide what to merge, both of which increase accuracy.
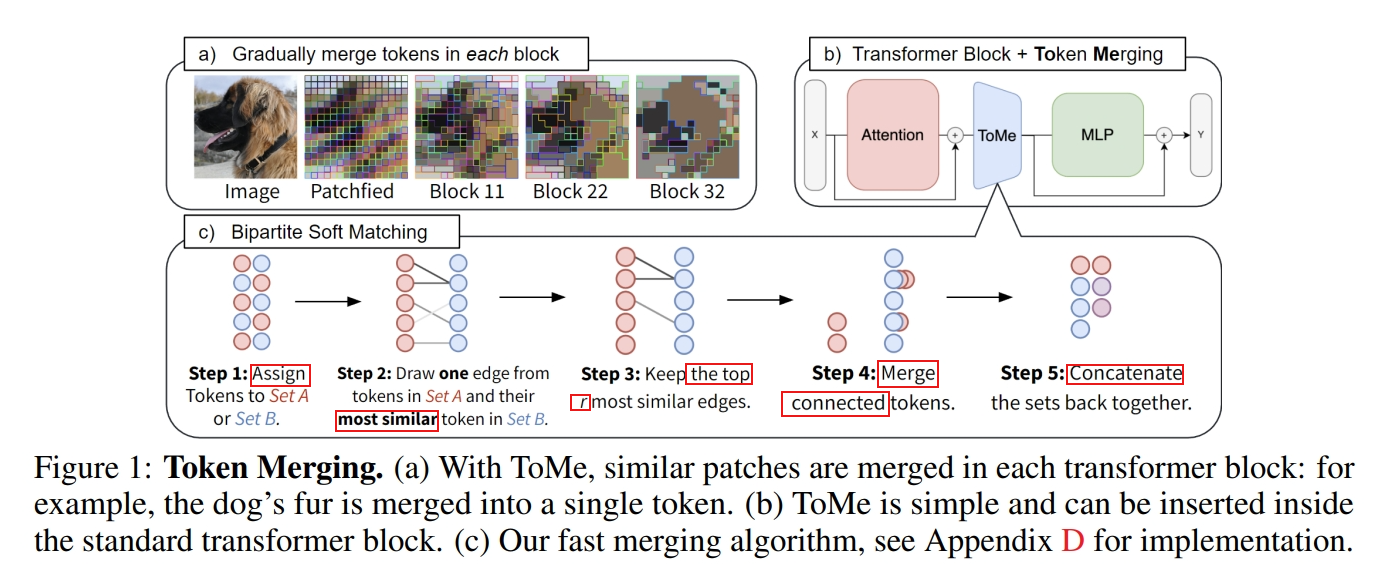
Token Similarity
Before merging similar tokens, we must first define what “similar” means.
Luckily, transformers natively solve this problem with QKV self-attention (Vaswani et al., 2017). Specifically, the keys (K) already summarize the information contained in each token for use in dot product similarity.
Thus, we use a dot product similarity metric (e.g., cosine similarity) between the keys of each token to determine which contain similar information.
Tracking Token Size
Once tokens are merged, they no longer represent one input patch. This can change the outcome of softmax attention: if we merge two tokens with the same key, that key has less effect in the softmax term.
We can fix this with a simple change, denoted proportional attention:
where s is a row vector containing the size of each token (number of patches the token represents). This performs the same operation as if you’d have s copies of the key. We also need to weight tokens by s any time they would be aggregated, like when merging tokens.