EagleEye: Fast Sub-net Evaluation for Efficient Neural Network Pruning
Abstract
Introduction
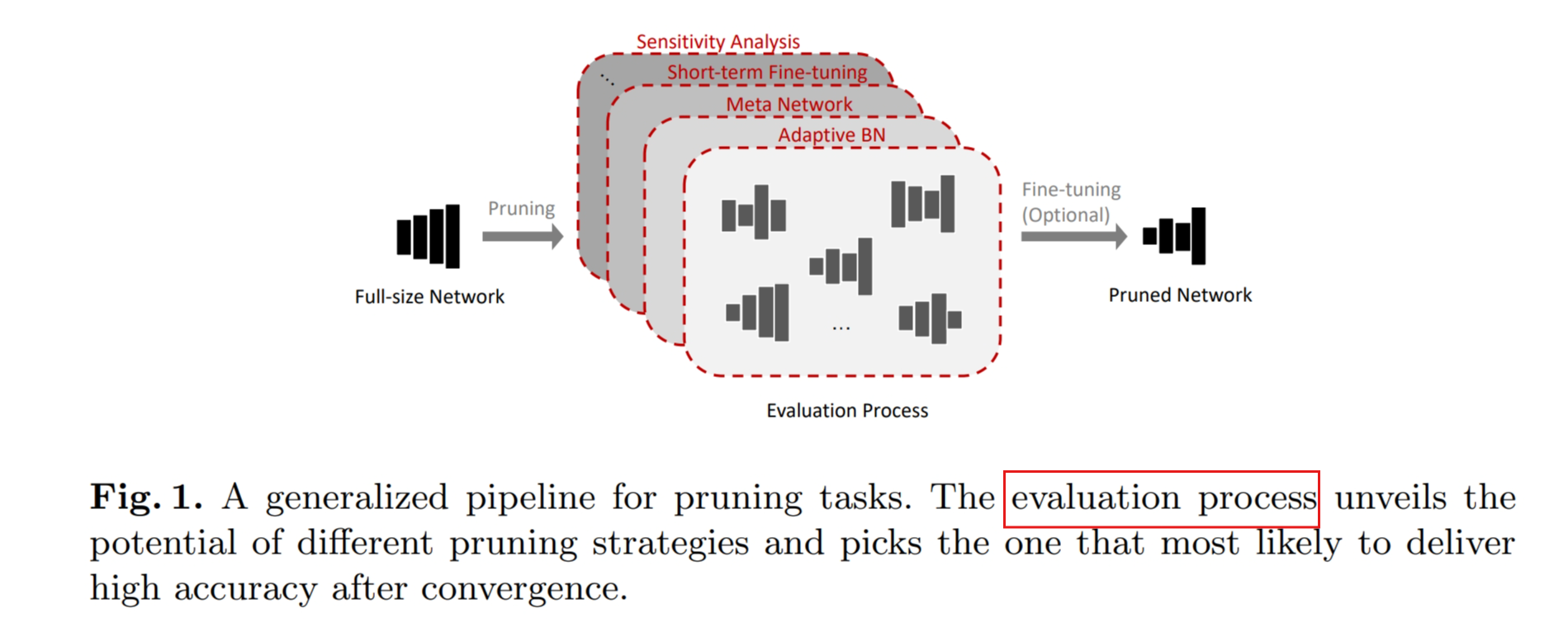
However, we found that the evaluation methods in existing works are suboptimal. Concretely, they are either inaccurate or complicated.
AutoML for Model Compression and Acceleration on Mobile Devices via reinforcement learning
To our knowledge, we are the first to introduce correlation-based analysis for sub-net selection in pruning task.
Moreover, we demonstrate that the reason such evaluation is inaccurate is the use of suboptimal statistical values for Batch Normalization (BN) layers.
In this work, we use a so-called adaptive BN technique to fix the issue and effectively reach a higher correlation for our proposed evaluation process.
NetAdapt - greedy method based
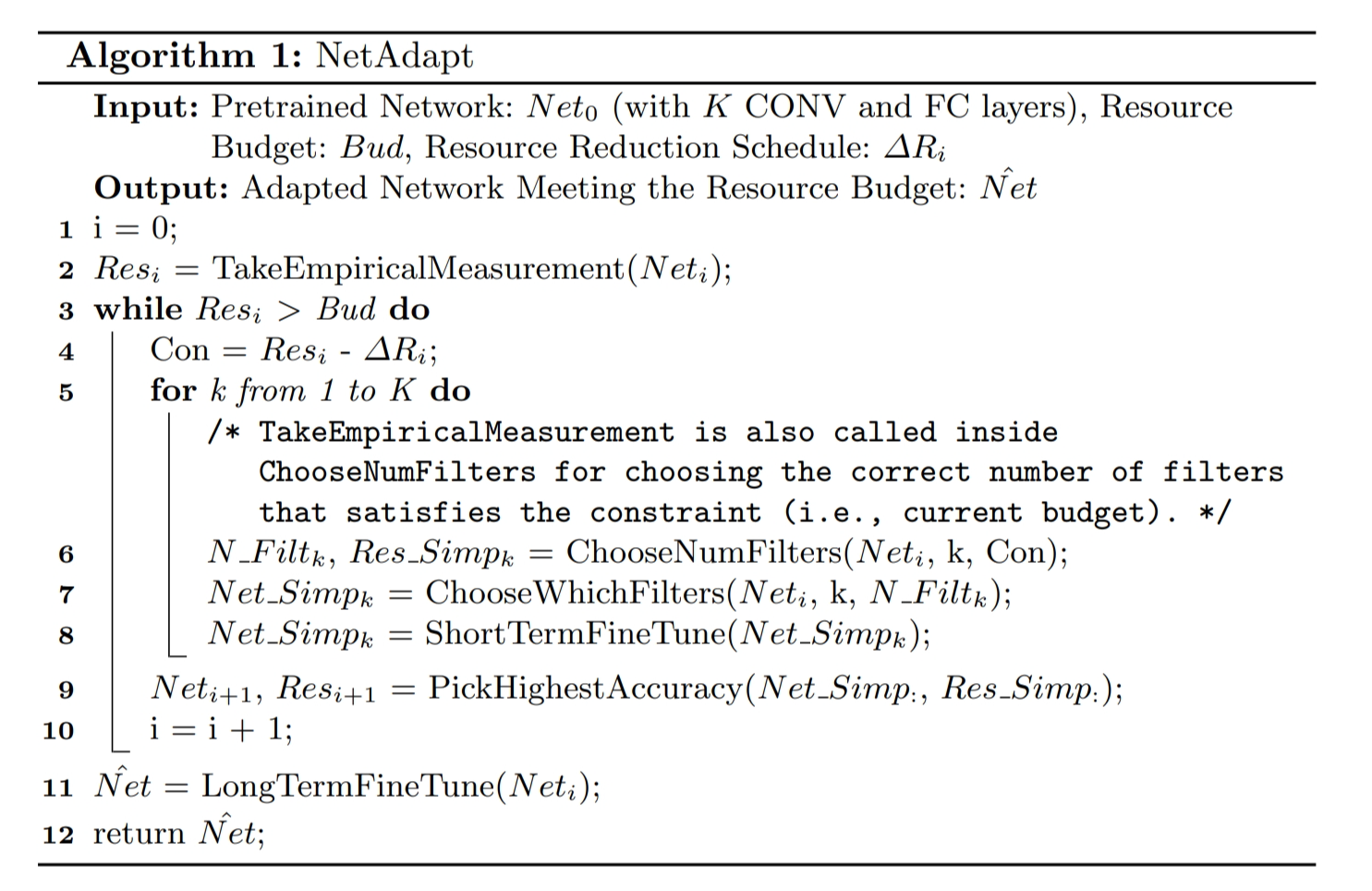
Introduces group-LASSO to introduce sparsity of the kernels
directly leverage the γ parameters in BN layers as the scaling factors
Towards Optimal Structured CNN Pruning via Generative Adversarial Learning
Methodology
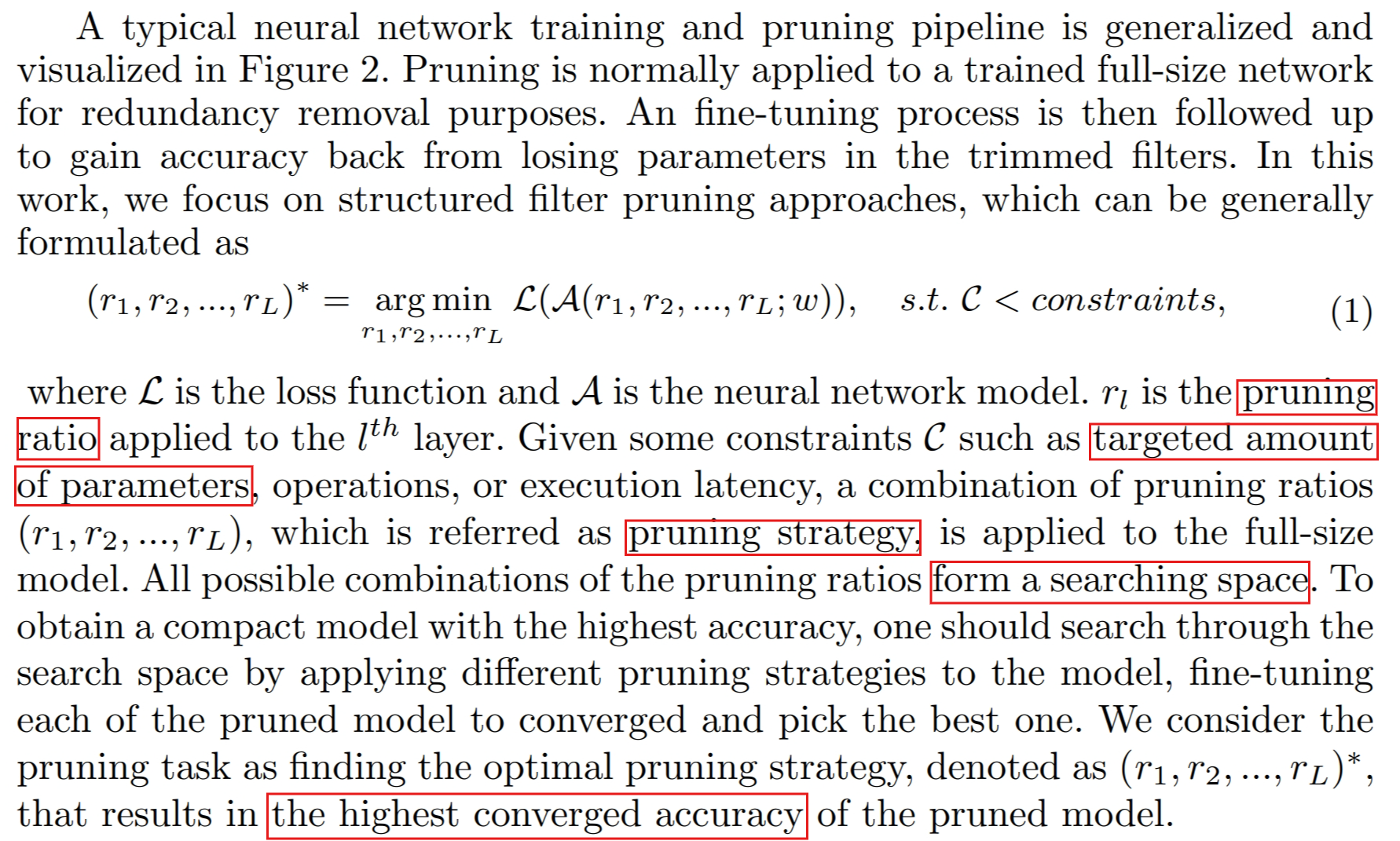
Different searching methods have been applied in previous work to find the optimal pruning strategy, such as greedy algorithm [26,28], RL [7], and evolutionary algorithm [20]. All of these methods are guided by the evaluation results of the pruning strategies.
Motivation
In many published approaches [7,13,19] in this domain, pruning candidates directly compare with each other in terms of evaluation accuracy. The subnets with higher evaluation accuracy are selected and expected to also deliver high accuracy after fine-tuning. However, such intention cannot be necessarily achieved as we notice the subnets perform poorly if directly used to do inference.
why removal to filters, especially considered as unimportant filters, can cause such noticeable accuracy degradation, although the pruning rates are random?
how strongly the low-range accuracy is positively correlated to the final converged accuracy
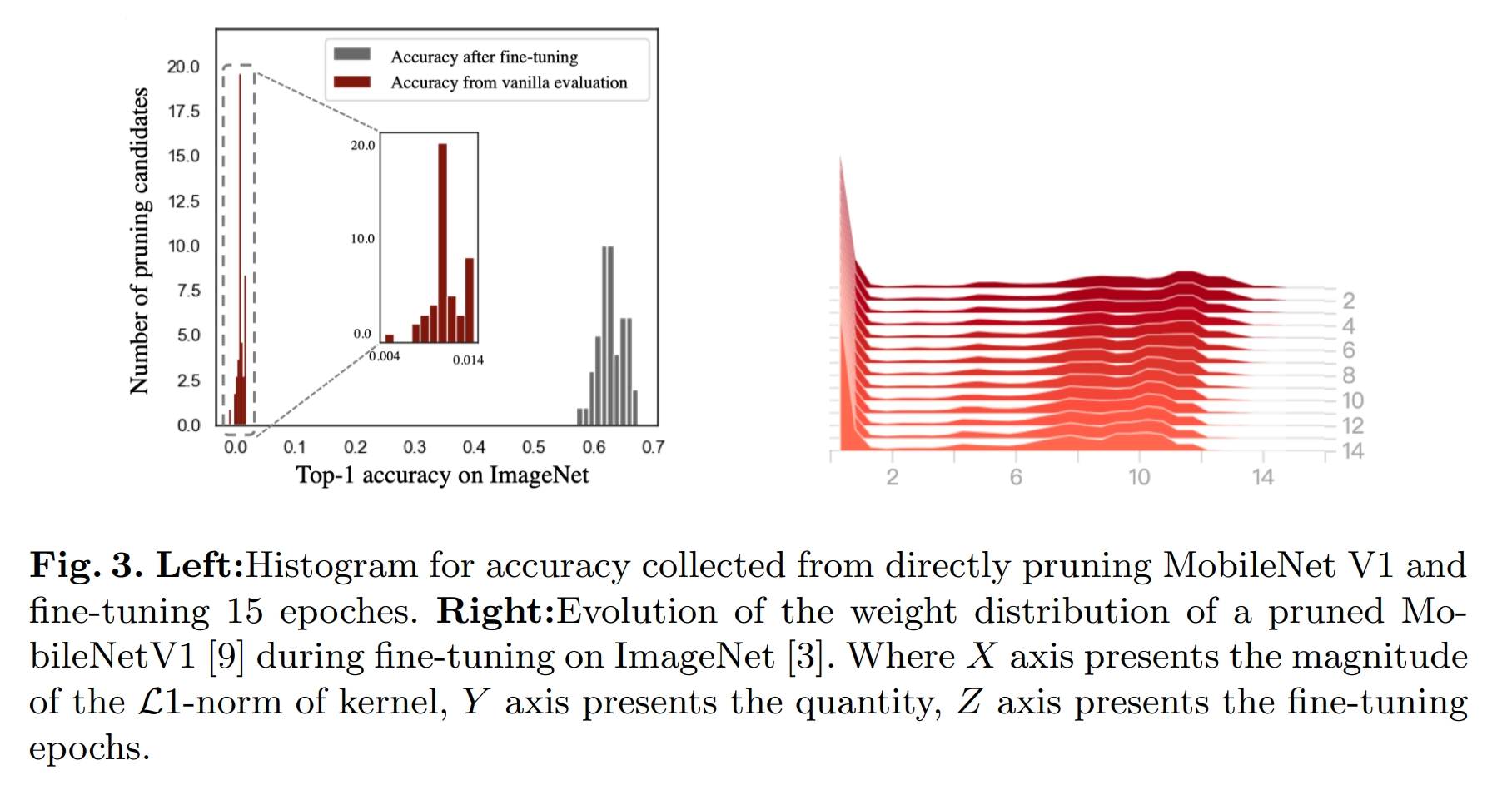
Figure 3 right shows that it might not be the weights that mess up the accuracy at the evaluation stage as only a gentle shift in weight distribution is observed during fine-tuning, but the delivered inference accuracy is very different.
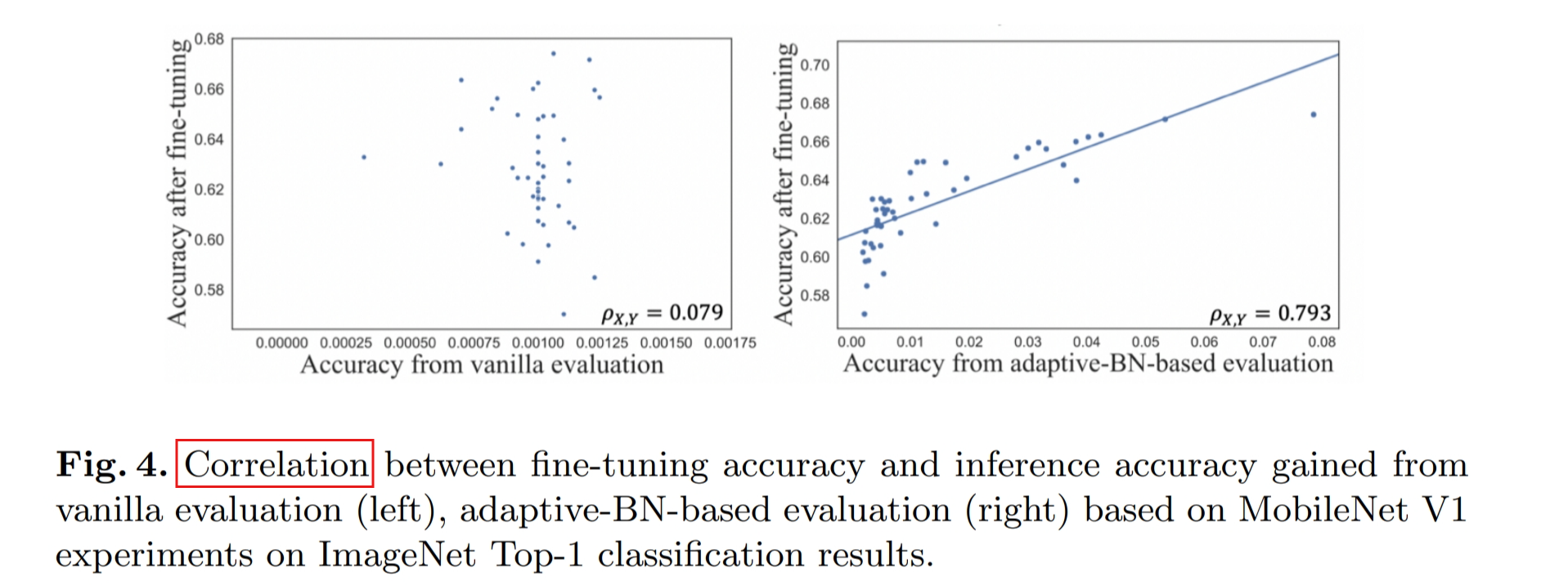
So the layer-wise feature map data are also affected by the changed model dimensions. However, vanilla evaluation still uses Batch Normalization (BN) inherited from the full-size model. The outdated statistical values of BN layers eventually drag down the evaluation accuracy to a surprisingly low range. And, more importantly, break the correlation between evaluation accuracy and the final converged accuracy of the pruning candidates in the strategy-searching space.
BN Basics
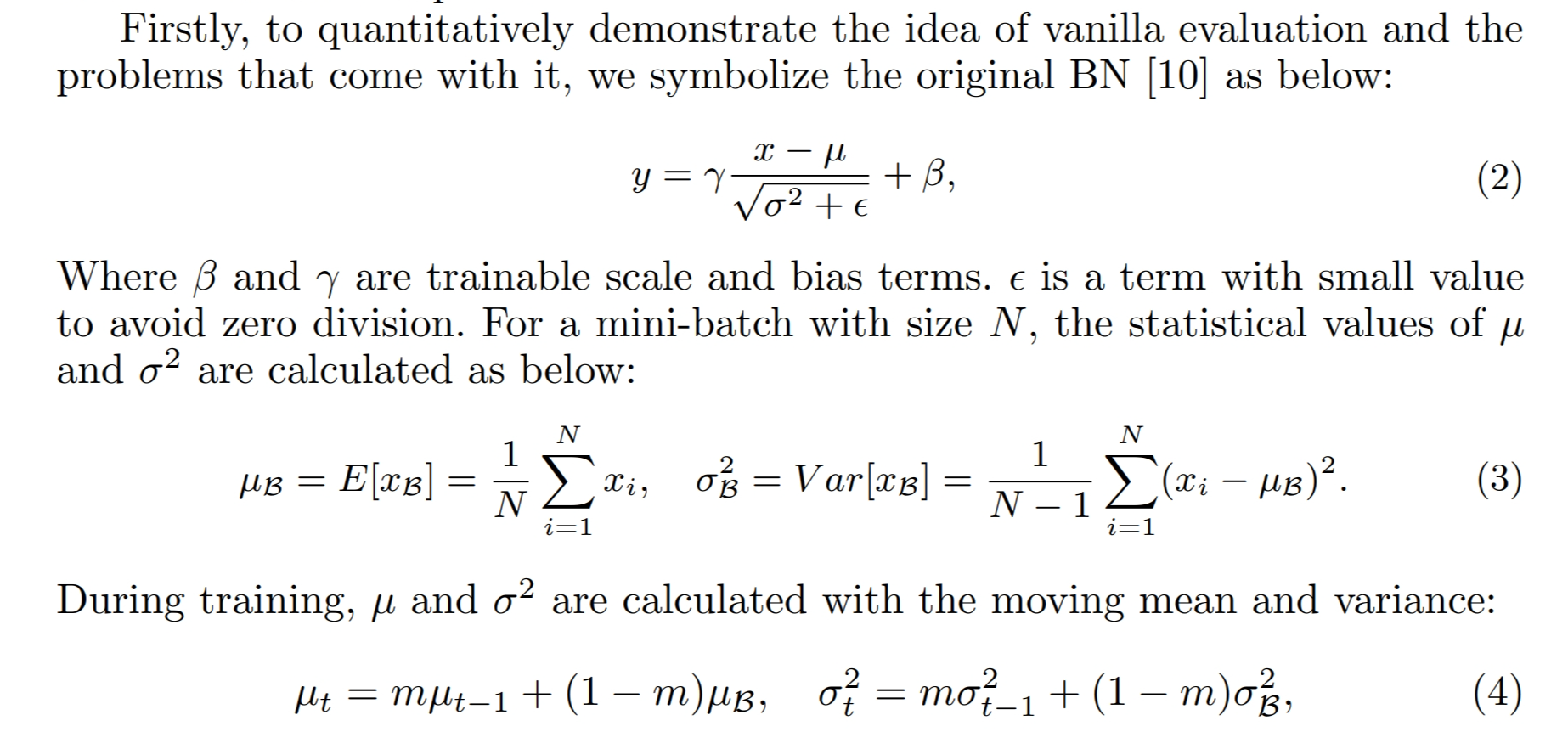
Adaptive Batch Normalization
If the global BN statistics are out-dated to the subnets, we should re-calculate µ_T and σ^2_T with adaptive values by conducting a few iterations of inference on part of the training set, which essentially adapts the BN statistical values to the pruned network connections. Concretely, we freeze all the network parameters while resetting the moving average statistics.
EagleEye pruning algorithm
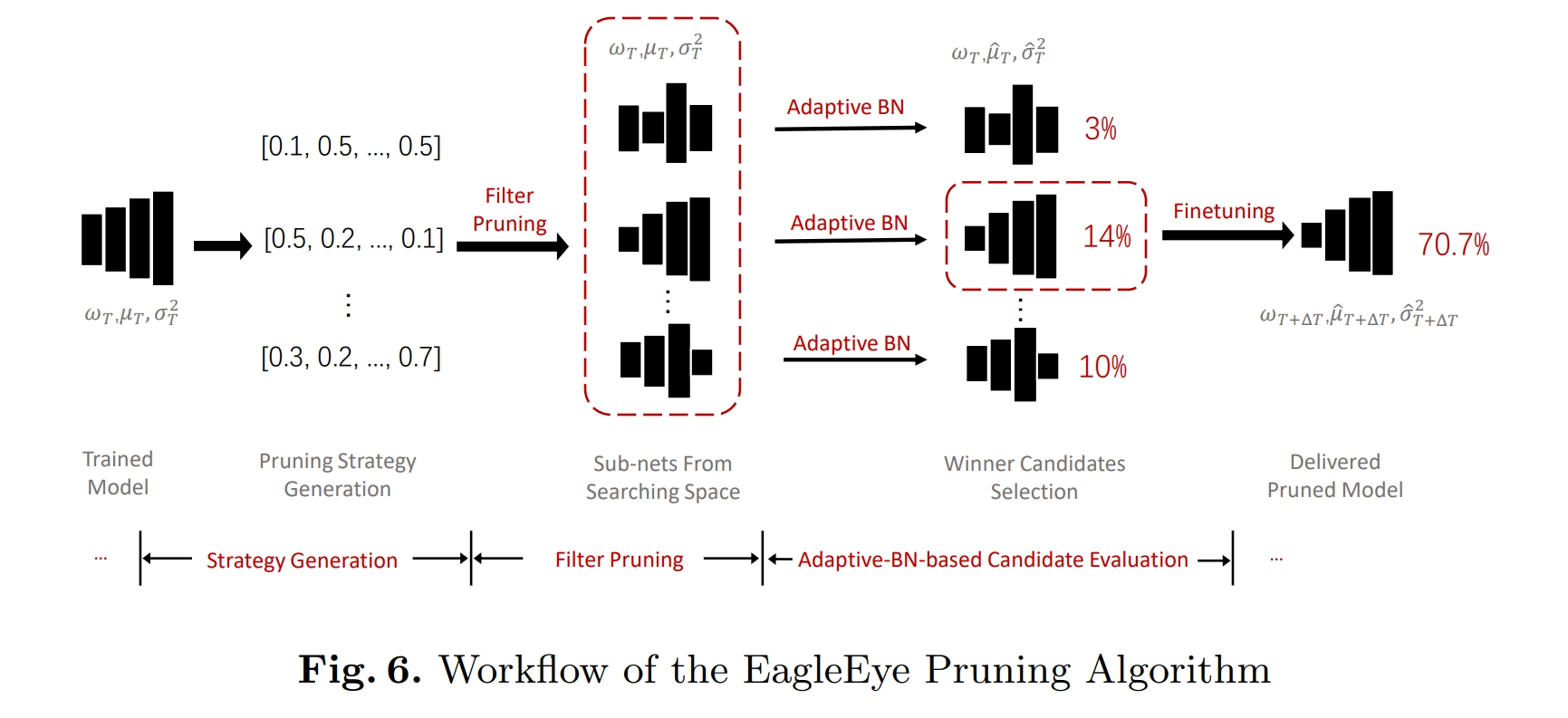
Strategy generation
Concretely, it randomly samples L real numbers from a given range [0, R] to form a pruning strategy, where r_l denotes the pruning ratio for the l th layer.